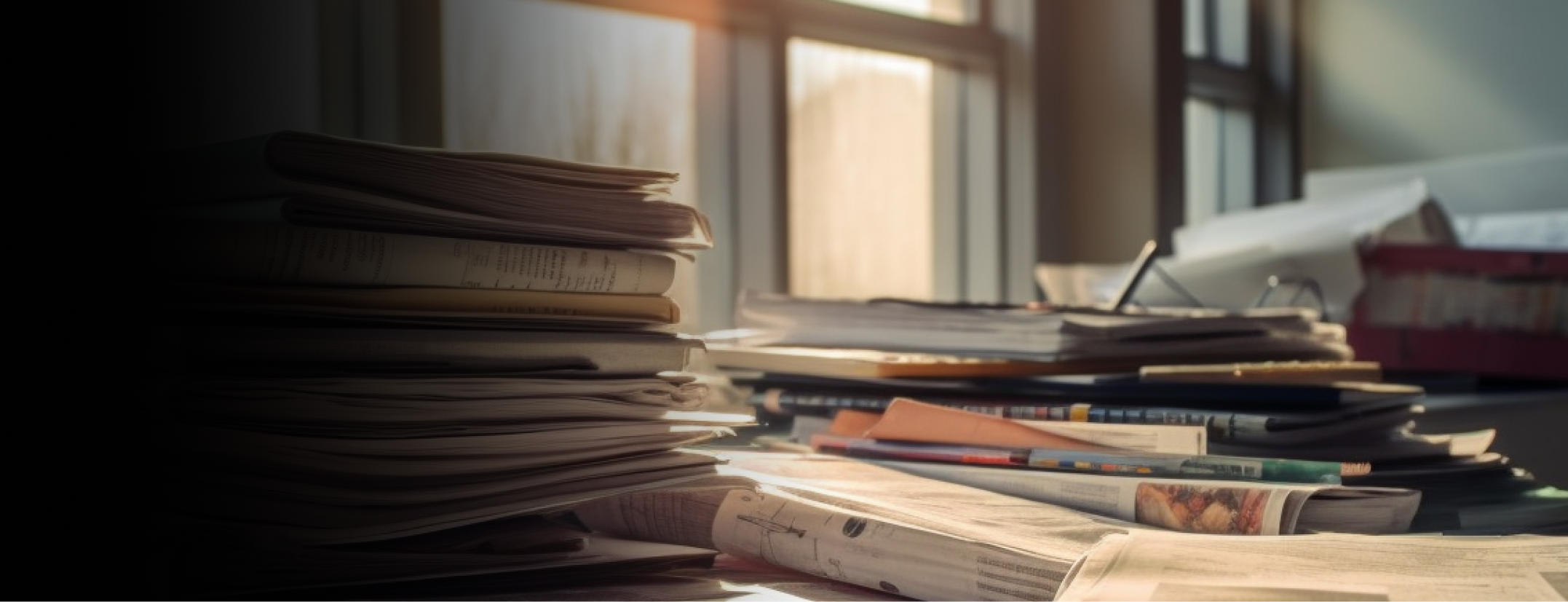
Research
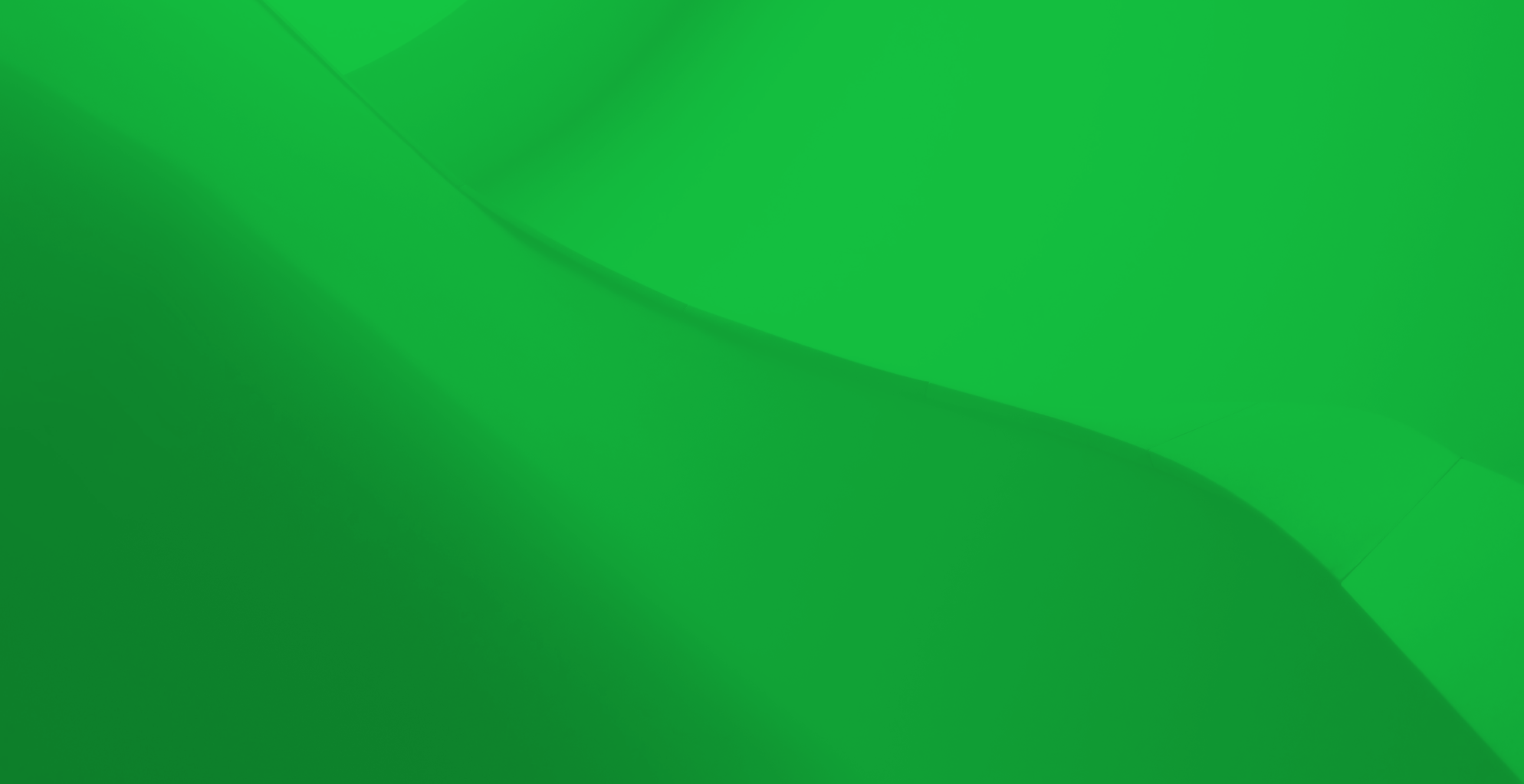
We focus our research on how to make foundation models safe enough for healthcare.
Polaris: A Safety-focused LLM Constellation Architecture for Healthcare
March 18, 2024 | 10 min read
Posted by Subhabrata Mukherjee, PhD and Paul Gamble, MD Hippocratic AI
The Healthcare Staffing Crisis
One of the most pressing challenges for the US Healthcare system today is the shortage of healthcare workers. The American Hospital Association has called the current shortage a national emergency, with many considering the staffing shortage the nation’s top patient safety concern [1]. According to a study by the Department of Health and Human Services, an astonishing 16.7% of hospitals anticipated a critical staffing shortage in 2023 [2]. Furthermore, the U.S. Bureau of Labor Statistics estimates a requirement of over 200,000 nurses each year until 2031 [3].
This, coupled with a growing elderly population that is expected to exceed 90 million by 2050 [4], inevitably worsens this gap in the supply and demand of our healthcare workforce, heightening concerns around patient safety and access to care. This concern has led to a surge in interest in using Generative AI for workflow optimization, such as inbox automation, EHR summarization, or ambient listening, to reduce documentation burden and burnout on healthcare workers. These solutions focus on improving productivity for healthcare workers. While valuable, their scope of impact is necessarily limited; even a 50% improvement in efficiency would not address the staffing shortages listed above.
Introducing Autonomous Healthcare Agents for Patient-facing Voice Conversations
To meaningfully address this national staffing crisis, we need a solution that can actually increase staffing. Our generative AI healthcare agents work fully autonomously, or in auto-pilot to perform non-diagnostic, patient-facing tasks typically performed by nurses, medical assistants, social workers, and nutritionists. Our AI agents are optimized for natural language, voice-based interaction, as speech remains the most effective medium for conveying nuance, building rapport and building trust, all of which are necessary for effective communication in a healthcare setting. However, building trust and rapport through voice requires a system that handles many complex issues, such as response lengths, audio quality, pauses, interruptions, and background noise. Further, healthcare conversations are exceedingly complex, typically purpose driven (i.e., there is specific information that must be elicited or conveyed) and require a high-level of accuracy. Thus, autonomous voice agents for healthcare conversations must have a robust safety infrastructure to address the inadequacies of current general purpose LLMs. Although the challenges are many, safe, autonomous generative AI healthcare agents can effectively address the healthcare staffing crisis. By serving as force multipliers, they can free up human healthcare workers to practice at the top of their license, at the bedside, and with patients that have the most acute needs.
At Hippocratic AI, our goal is to build these specialist agents. We are on a mission to ensure that access to high quality care is not limited by staffing constraints and workforce fatigue. We aim to create an era of super staffing, and redefine the standard of care in healthcare.
Figure 1. Overview of our architecture, comprising of the Automatic Speech Recognition (ASR) for speech transcription, Polaris for processing the textual utterances, and Text-To-Speech (TTS) for the audio output. The constellation within Polaris contains a primary LLM agent driving the conversation, and several specialist LLM agents providing task-specific context to it.
Our Safety-focused Constellation Architecture
To achieve our goal, we built Polaris, a novel constellation architecture with multiple specialized healthcare LLMs working in unison. We found this architecture allowed for accurate medical reasoning, fact-checking, and the avoidance of hallucinations, while maintaining a natural conversation with patients. Safety is our North Star. We name our system after Polaris, a star in the northern circumpolar constellation of Ursa Minor, currently designated as the North Star.
Polaris comprises a primary conversational agent (70B - 100B parameters) supported by several specialist agents totaling over one trillion parameters for the constellation. This primary agent is aligned to adopt a human-like conversational approach, exhibiting empathy and building rapport and trust. The primary agent has also been trained to follow a care protocol (i.e., a checklist of tasks that must be completed) and to track its progress in completing the required tasks.
The specialist agents are optimized for healthcare tasks. These include OTC toxicity detection, prescription adherence, lab reference range identification, and others shown in the figure above. The specialist agents “listen” to the conversation and guide the primary model if the discussion enters the specialist model’s domain. For example, if the patient asks how many Tylenol they are allowed to take, the medication specialist agent performs prescription adherence verification to “whisper” the answer to the primary agent; the agent has been trained on OTC drug specifications and can thus accurately recall the manufacturer’s dosage instructions. A key specialist model is our “human intervention specialist”. This model is trained to detect unsafe medical situations – e.g, a patient describing life-threatening symptoms – and promptly transfer the conversation to a human nurse.
We developed custom training protocols for conversational alignment using organic healthcare conversations and simulated conversations between patient actors and U.S. Licensed human nurses; the conversations were reviewed by U.S. licensed clinicians for our unique version of reinforcement learning with human feedback (RLHF). In addition, the system was trained on proprietary data including clinical care plans, healthcare regulatory documents, medical manuals, drug databases, and other high-quality medical reasoning documents.
Figure 2. Overview of our training framework for Polaris with registered nurses, patient actors, and LLM agents in the loop.
We show a visual depiction of our training framework in the above figure. The specialized nature of Polaris and patient-focused AI conversations require specialized alignment. We developed an iterative training protocol as follows:
Instruction-tuning on a large collection of healthcare conversations and dialog data
Conversation tuning of the primary agent on simulated conversations between patient actors and registered nurses on clinical scripts that teach the primary agent how to establish trust, rapport and empathy with patients while following complex care protocols
Agent tuning to teach the primary agent and specialist agents to coordinate with each other and resolve any task ambiguity. This alignment is performed on synthetic conversations between patient actors and the primary agent aided by the specialist agents of the constellation.
Conversation and Agent tuning were performed iteratively with self-training the primary agent to allow generalization to diverse clinical scripts and conditions and mitigate exposure bias.
Finally, human nurses played the role of patients and talked to the primary agent guided by the support agents. The nurses provide fine-grained conversational feedback on multiple dimensions including safety, bedside manners and knowledge as well as providing re-writes for bad responses used for RLHF.
Evaluation Protocol and Ground-breaking Results
We developed and are conducting a novel three phase safety evaluation of Polaris.
Phase one involved U.S. licensed physicians and nurses ensuring the agent completed all critical checklist items for a given use case. For this phase, we were primarily focused on conversational speed and flow, task completion, and factual accuracy.
In phase two testing, we assessed the integrated overall performance of Polaris through a series of calls between a fictional patient and our AI Agent. As a control, we conducted similar calls between a fictional patient and a separately recruited human nurse (U.S. Licensed). In each call, the fictional patients were played by patient actors, human nurses (U.S. licensed), or human physicians (U.S. licensed). Prior to each call, the study participant was given a background and medical history for the fictional patient. Our AI assistants and the human nurses (for the control group) were given that same background and medical history, as well as a detailed clinical history and call objectives. After each call, the participants answered a series of questions, evaluating the AI agent on a variety of dimensions including bedside manner (e.g., empathy, trust, rapport), medical safety, medical knowledge, patient education, clinical readiness and overall conversation quality.
Participants identifying as a U.S. licensed physician or nurse were required to provide their licensing credentials and other identifying information, which we verified against publicly available license databases. The participating nurses and physicians had a range of experience levels, came from a variety of specializations, and work(ed) at different U.S. institutions.
In addition, we assessed the performance of the specialist agents in isolation. We provided the various specialist agents a set of test cases consisting of fixed statements (clinical scenarios) and follow-up instructions. The agents were evaluated on the appropriateness and correctness of their responses.
The results of our phase 2 test are summarized below. Impressively, on subjective criteria, our study participants rated our AI agent on par with U.S. Licensed nurses on multiple dimensions. On objective criteria, our medium-size AI agents significantly outperformed much larger general-purpose LLMs like GPT-4 in medical accuracy and safety.
Subjective measures (measured against human nurses):
Figure 3. Comparative evaluation between U.S. Licensed nurses and our AI on bedside manner (e.g., empathy, trust, rapport), medical safety, medical knowledge, patient education, clinical readiness and overall conversation quality. Overall, our AI was rated strikingly close to human nurse performance, and was even found to outperform them on some key dimensions
Table 1. Subjective evaluation for Polaris by U.S. Licensed nurses and U.S. Licensed physicians on various perspectives such as bedside manners, clinical readiness, patient education, medical knowledge and medical safety compared against U.S. Licensed nurses.
Table 2. Capability performance for different specialist agents from Polaris compared against LLaMA-2 70B Chat and GPT-4.
Examples of our constellation system at work:
Case study 1 (at a support agent level): A patient mentions a blood sugar of 104. The lab specialist agent evaluates the value and clarifies whether this is taken while fasting or after a meal (postprandial). When told it was a fasting value, the lab agent informs the patient that the value is within the expected reference range for fasting blood sugar. Following this the lab agent retrieves the patient’s prior blood sugar readings and performs a trend analysis, providing praise on improvements and encouragement where needed. This reminds the patient that they have a question about their diabetes medication dose, specifically for Metformin. The medication specialist agent informs the patient about their prescribed dose, checks their adherence to dose, timing and frequency and explains what Metformin does. The EHR specialist agent documents the new blood sugar range for the human care team..
Case Study 2 (at a capability level): A patient mentions taking three tablets of a medication they incorrectly pronounce ‘Benadodril’. Our medication specialist agent employs its drug misidentification capability to recognize that this is not a real medication, and that there are two commonly confused but similar-sounding medications, Benadryl and Benazepril. Using a variety of techniques, the agent clarifies that the patient is referring to Benadryl. It further performs condition-specific disallowed OTC (over-the-counter medication) verification, for which it has been trained on OTC drug labels, to check that Benadryl is not prohibited based on the patient's medical conditions. Finally, it performs an OTC toxicity verification that the patient has not exceeded the manufacturer's daily maximum dosage.
Conclusion
We are now moving to phase three testing, which requires extensive evaluation to be completed by at least 5,000 licensed nurses and 500 licensed physicians, as well as by our health system and digital health partners. To the best of our knowledge, we are the first to conduct such an extensive safety assessment of any Generative AI technology for real-life healthcare deployment.
We foresee a promising future for AI agents to improve healthcare by filling a large portion of the staffing gap. As we continue to push the boundaries and overcome challenges, our goal remains to provide scalable and safe systems that alleviate the burden on human health care providers and improve patient satisfaction, health care access, and health outcomes.
References